ML/AI System: Architectural Patterns
Whether you're an ML engineer, software architect, or technical leader, this guide provides you with the knowledge to build ML/AI systems that handle real-world challenges and deliver consistent, high-quality results.
About the author: VP of Engineering, Program Manager, Coach, and Founder, specializing in career coaching, behavioural and system design prep. Book time with them here.
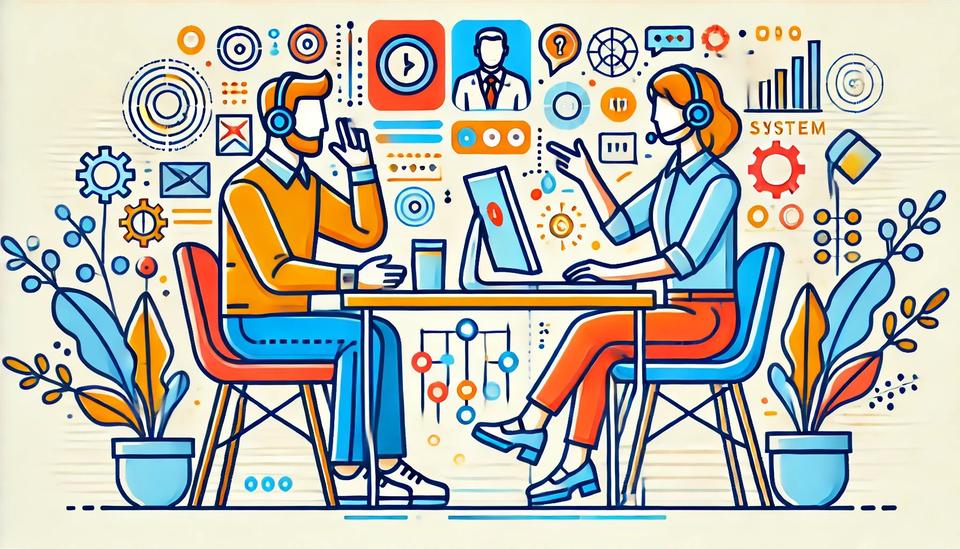
As the adoption of machine learning (ML) and artificial intelligence (AI) continues to grow across industries, the need for robust, scalable, and well-designed ML/AI systems has become increasingly crucial. Gone are the days when ML/AI were relegated to standalone, experimental projects. Today, these technologies are mission-critical components integrated into the core of many applications and systems. Ensuring the reliability, performance, and maintainability of these ML/AI systems is paramount to their success.
ML/AI:
1. How LinkedIn Detects Spam Content:
LinkedIn employs a sophisticated system for detecting spam posts and updates on their platform. This system leverages a combination of natural language processing (NLP) techniques to analyze the content of posts, machine learning models to identify patterns indicative of spam, and heuristics to flag suspicious behavior. Additionally, human moderation workflows are in place to review and take action on flagged content, ensuring that legitimate posts are not incorrectly marked as spam.
For an in-depth look into how these systems work, including specific examples and case studies, read the full article here.
2. How Spotify Generates Ad Content at Scale:
Examines Spotify’s infrastructure for dynamically generating personalized audio/visual ad content at scale across devices/platforms. Covers areas like content management, ad targeting systems, and optimizations.
For a detailed exploration of this topic, read the full article here.
3. How OpenAI Trained ChatGPT:
Provides insights into the training process and architecture behind OpenAI’s ChatGPT language model, including data curation, model architectures, distributed training approaches, and techniques like constitutional AI.
For a comprehensive look into these aspects, read the full article here.
4. How Airbnb Discovers What Users Like:
Covers Airbnb’s recommendation systems for surfacing relevant listings, experiences, and content tailored to each user’s preferences through techniques like collaborative filtering, matrix factorization, and deep learning models.
To gain deeper insights, read the full article here.
5. How Microsoft diagnoses production issues with LLMs:
Explores Microsoft’s approach to monitoring, debugging, and root cause analysis for large language models in production using observability tools, distributed tracing, canary deployments, and other strategies.
Uncover profound insights by exploring the full article here.
How Coaches Can Help
Developing robust ML/AI systems requires expertise across a range of domains, including software engineering, data science, operations, and more. Engaging experienced coaches and consultants can be invaluable in navigating the complexities of ML/AI architecture and design. Coaches can provide guidance on:
- Defining the right architectural patterns and design principles for the problem domain
- Identifying potential failure modes and building resilience and observability
- Optimizing model training, deployment, and lifecycle management workflows
- Incorporating security, privacy, and responsible AI best practices
- Mentoring cross-functional teams to foster collaborative problem-solving
- Sharing lessons learned from previous large-scale ML/AI deployments
By tapping into the knowledge and experience of seasoned ML/AI coaches, organizations can accelerate their journey towards building reliable, scalable, and maintainable systems that unlock the full potential of these transformative technologies.
Conclusion
As ML/AI become increasingly integral to modern applications and systems, the need for robust, scalable, and well-designed architectures has never been more critical. The case studies outlined in this blog post showcase how leading organizations are tackling this challenge, from spam detection to dynamic ad generation to large language model deployments. By learning from these real-world examples and leveraging the guidance of experienced ML/AI coaches, organizations can build the resilient, adaptable, and maintainable systems required to unlock the full potential of these transformative technologies.
About the author: Andrea Della Corte
VP of Engineering, Program Manager, Coach, and Founder, specializing in career coaching, behavioural and system design prep. Book time with them here.